The ability to form informed opinions is key to success in today's fast-moving business world. Whereas organizations are fighting the battle of data volumes every day, the problem is not in collecting the data, but it lies in converting it into meaningful results. Artificial intelligence changes choices made in how the company does things in this situation. Companies can discover complicated data-driven decision-making sets, find the samples, and forecast future trends with unprecedented speed and velocity by using AI findings.
AI transforms traditional data-driven marketing into a dynamic process that drives strategic initiatives. Artificial Intelligence enables organizations to be more effective and efficient through the automation of repetitive operations, anticipating user behavior. As we delve into what artificial intelligence insight is and how one can implement data-driven decision-making, we will find the must-haves that make AI-driven marketing disruptive. We are also going to talk about the benefits that using artificial intelligence insight will have across a wide range of sectors and provide important resources to help enhance your company's analytical skills.
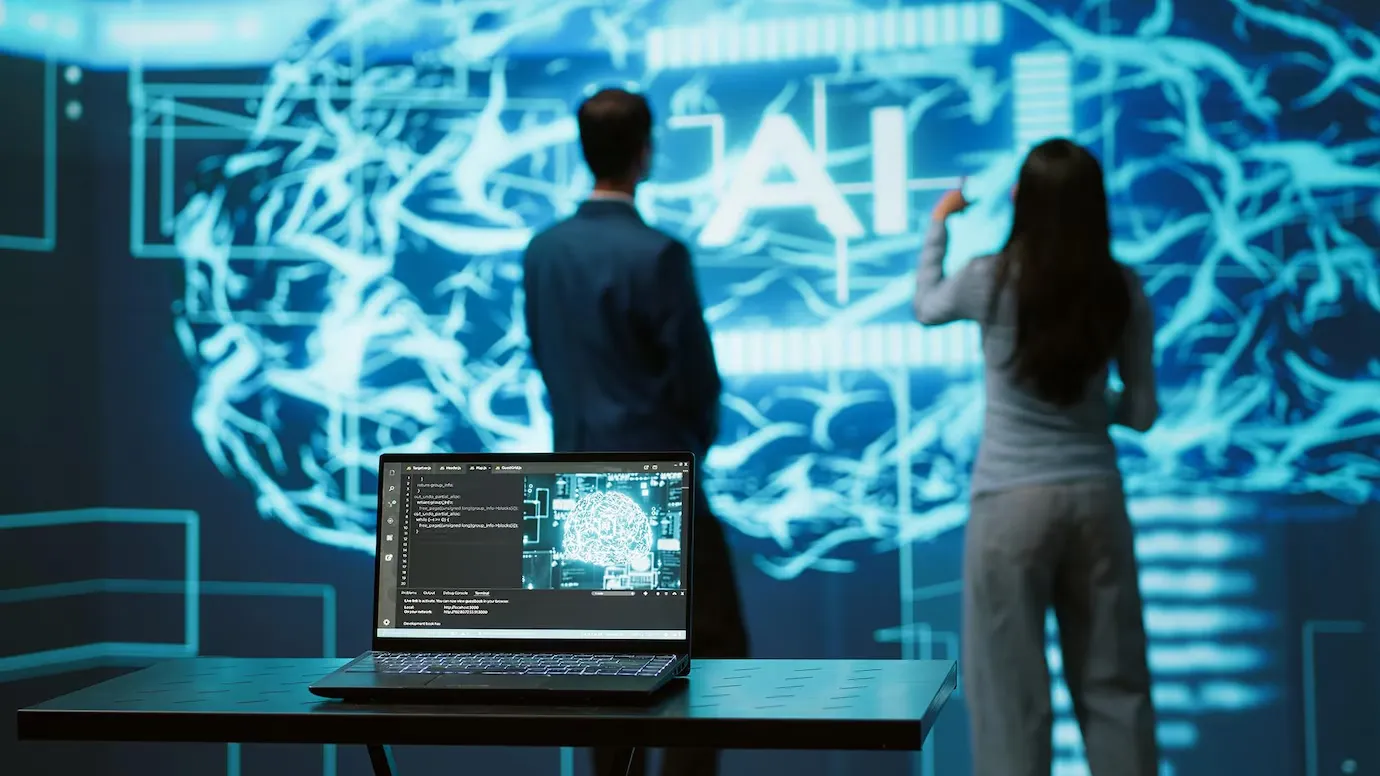
This article gives insight into artificial intelligence and its unlocking and unleashing of whole opportunities to make data-based AI decisions that allow businesses to stay competitive in a landscape that gets even more competitive by the minute.
What are AI insights
AI insights dualmedia solutions combine multiple data streams to provide comprehensive business intelligence across digital and traditional channels. As the world continues to get more and more busy, business is right behind. Business companies are constantly looking for ways to overcome the competition. Artificial Intelligence (AI) is one of the strongest tools in their disposal. With the ability to deal with vast amounts of data, AI has revealed business companies to unprecedented levels of getting insights, taking well-informed decisions, and optimizing their operation. With AI, business organizations are able to access a competitive advantage that will transform everything from customer service to advertising. AI is transforming how business organizations function by enabling them to sort and analyze data in a better way.
With machine learning and automation, AI applications are able to identify patterns from past information that would otherwise go unnoticed. AI-driven business insights enable companies to uncover hidden patterns in customer behavior and market trends that would otherwise go unnoticed. This allows businesses to make decisions based on solid facts and not speculations or assumptions. From predicting future trends, buying behavior patterns, to optimizing operations procedures, AI provides businesses with a whole lot of useful information that can guide them to make intelligent decisions. One of the largest benefits of AI is that it helps businesses discover more about their customers. With analysis of customer engagement data, AI can identify trends and leanings that help businesses tailor their products, services, and ad campaigns based on customers' needs. By leveraging an Ideal Customer Profile Generator, businesses can refine their marketing strategies based on AI-driven insights, ensuring they target the most relevant audiences with precision. This can lead to higher levels of customer satisfaction and loyalty since businesses have a better chance of offering more personalized and relevant experiences.
Besides creating customer relationships, AI can even unveil the secret to enhancing business processes.
By implementing automatic processes and streamlining workflows, AI can free up valuable resources and time that can be used for higher-strategic pursuits. For example, AI-driven chatbots can aid in resolving customer inquiries, while predictive analytics can assist businesses in predicting demand and having improved inventory management. These technologies not only make processes easier but also cut costs and increase productivity. AI is also fundamentally changing another sector, the sector of data-driven decision-making.
Before, most corporations previously relied on instincts or experience to make decisions. But with technology, corporations are now able to use recent information and better analytics that could give a better picture of what is happening in the market. For instance, AI may go through sales histories and predict trends of what product will be popular in the future, and therefore enable firms to reorder marketing and inventory decisions accordingly. This ability to predict what comes next is itself an organisational revolution to keep pace with and lead the times. Besides that, AI is also a great buddy in terms of campaign optimization for marketing. With the help of AI, companies are able to come up with effective target marketing plans that have greater chances of communicating with their targeted audience through analyzing consumer behavior and preferences. Using a Marketing Strategy Generator, companies can transform raw data into actionable plans, optimizing campaigns and resource allocation for maximum efficiency. Right from targeted promotions to recommendations of customization, AI helps companies converse with consumers in a more one-to-one basis, which later translates into more engagement and conversion.
As businesses continue to produce more data and face more advanced problems, AI will only become even more central.
Those businesses that are able to harness the power of AI and integrate it into their decision-making will be primed to flourish in the years to come. By taking data-driven decisions and embracing the power of AI, not only do organizations enhance their operations but also strengthen their bond with their customers, develop improved products, and tackle the constantly changing dynamics of the market with ease. In general, AI is revolutionizing the business practice by giving firms effective data analysis, automation, and decision-making power. Firms that will want to remain competitive in the next few years will be required to utilize AI and leverage its power. Through the utilization of the use of AI in deriving insights, building customer relationships, automating work, and optimizing ad campaigns, firms can set themselves up for long-term success in the age of information. Modern businesses derive insights with artificial intelligence by processing vast datasets in real-time to support agile decision-making.
Understanding Data-Driven Marketing
Data-driven marketing is the practice of basing marketing choices on facts and replacing gut feeling and intuition with extended power data driven decision making analysis. Artificial Intelligence (AI) can play a vital part, by making it possible to automate data collection, preparation, and analysis. This enables firms to send the appropriate messages to the most pertinent audiences at the appropriate times.
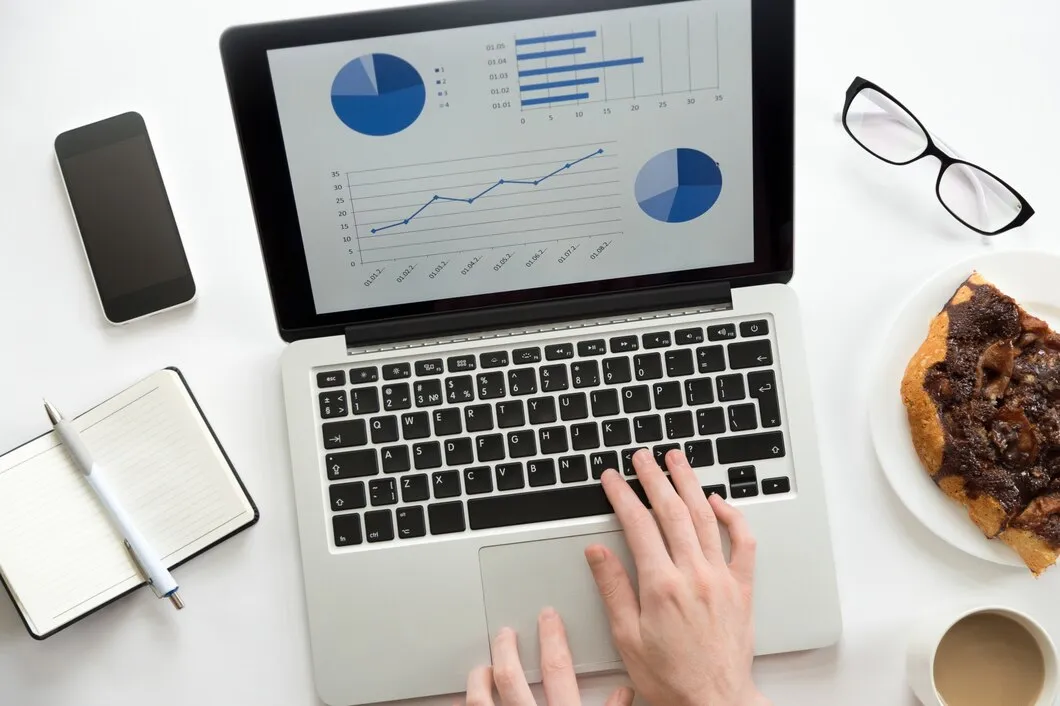
Step 1: Data Collection
The collection of different information from diverse sources, including websites, social media, consumer interactions, and sales records, is the initial stage in data-driven marketing. This critical data diversity for creating a thorough grasp of customer insights AI tastes and habits. This procedure may be automated with AI techniques, guaranteeing precise and consistent data driven decision making collection. Businesses may save time and lower the possibility of human mistakes during data collecting by utilizing these tools.
Additionally, the data may be divided into two categories: stored data, which includes information that is simply arranged, such as customer names and emails, and unstructured data, which includes more complicated formats like posts on a social media site and user comments. Marketers may develop more efficient consumer strategies through a better understanding of consumer sentiments and trends via the analysis of both structured and unstructured data.
Step 2: Data Processing
Data cleaning, formatting, and arrangement are the steps that must follow the data collection event. Artificial intelligence (AI) algorithms can assure high-quality, reliable data for big data-driven decision making sets by eliminating duplicates, adding missing variables, and conversion of non-structured dates into a usable format. This crucial step guarantees that insight data analytics are based on the highest possible levels of data quality.
Firms can both maximize the worth of their poll responses and lower the likelihood of adverse outcomes in future research by precisely ordering their data. Data combination understanding of the pattern recognition and trends is easier with the well-structured data which may be found in other data driven decision making sets. Eventually, the time and effort put into data purging prepare a solid grounding for the strategic planning and decision-making process AI.
Step 3: Data Analysis
AI driven insights transform raw data into actionable intelligence, helping businesses optimize operations and customer experiences. Following analysis of data driven decision making for processing will identify tendencies, and reveal patterns and correlations. Massive datasets may be swiftly combed through by AI systems to find AI insight that conventional analytical techniques overlook.
AI may identify minute changes in customer insights AI patterns of behavior that analysts would miss by using sophisticated algorithms, which deepens the analysis. AI, for instance, may help retailers better identify and forecast buyer patterns and upcoming product needs in the best way to market tactics.
Furthermore, AI solutions enable highly segmented customers based on their preferences and shopping behavior for targeted marketing measures. In addition to improving consumer interaction, this capacity improves resource allocation and ensures that you maximize the return on your marketing decision making initiatives on investment.
Step 4: Visualizing The Data
AI applications & insights are revolutionizing industries from retail to healthcare by providing predictive analytics and personalized recommendations. Data visualization of findings from the data-driven decision-making analysis is the final stage of data-driven marketing. When done well, it means business enterprise can more easily comprehend complicated information and communicate ideas among teams. The comprehensive charts, graphs, and dashboards that AI technologies can provide enable decision-makers to quickly comprehend the most salient findings and to take action.
This in itself can highlight several trends or patterns that would otherwise have passed unnoticed by transforming raw data-driven decision making into visually pleasing representations. Moreover, these visualizations also create avenues for team members to go over the data together and hence come up with practical solutions; thus encouraging cooperative conversations.
At the end, effective data-driven decision making visualizations enhance understanding but also inspire participation and build confidence in the AI decision making process. AI-based operational insights help organizations streamline processes, reduce costs, and improve efficiency through continuous data monitoring.
Key Components of AI-Driven Marketing
To use AI appropriately for marketing, businesses need to understand the elements that constitute essential parts of AI-driven insight marketing processes. These ensure data-driven decisions on accuracy analysis and reliable, helpful discoveries.
Thus, businesses will better create specific actions on their strategy of entirely maximizing the AI; more personal touch between a company and clients' experiences; more convincing market powerhouse effort in AI marketing tool techniques. This in-depth understanding can even make any integration easy regarding adding this type of technology in the company's ongoing lawsuit processes, building and nurturing data decision making using an AI way.
Innovativeness and adaptability are the decisive factors for achieving long-term success in the digitally moving environment, and all such competencies help companies stay competitive in business.

Data Collection and Integration
First of all, easy collection and integration of AI for decision making from multiple sources act as the gateway to successful AI-driven insights marketing. The AI solutions may compile information from social media, CRM platforms, mobile applications, and websites to provide a complete view of client interactions. This connection assures accuracy and consistency of data for analysis that an organization needs in understanding consumer behavior and preferences.
Organizations can also identify patterns and AI insight that the combining of data from many sources can overlook. Moreover, a single dataset allows more precise segmentation and targeting, raising the efficacy of marketing decision-making tactics. This basic action, in the end, lays the foundation to leverage AI for boosting conversion rates and meaningful engagement.
Data Preprocessing
The preprocessing of data before analysis helps ensure product integrity and durability. Such preprocessing may include transformation, normalization, and cleansing of data. This important step takes out the errors and duplication that would distort the findings of any analysis.
Allow the integration to be smooth and comparable; pre-processing of data, collected from so many sources, requires standardization of format. These could be done automatically using AI, thus saving valuable time and securing the accuracy and reliability of the data used in any analysis for much more perceptive and useful insights.
Exploratory Data Analysis (EDA)
EDA is the process of looking at datasets and summarizing their key features, often with the help of some display methods. Using box plots, scatter plots, and histograms are other ways of conducting EDA, adding to the analyst's insight into the hidden patterns by offering AI with a view of the variability and distribution of data.
EDA helps to find out trends, detect anomalies, understand the distribution of data, and helps stakeholders to form theories and sharpen their questions for a more focused investigation in later stages. Companies can get quick insights at a high level into their data-driven decision-making and perform preliminary assessments by leveraging AI that automates EDA.
This can enhance the quality of AI insight that businesses derive from their computational biology algorithms in finding intricate links in the data, which may not be seen when done manually.
Feature Engineering
Function building refers to the process of constructing new functions-variables-from raw data-driven decision making in order to enhance the performance of predictive models. It entails the selection of the most relevant data properties that would contribute to enhancing the accuracy of the model.
With proper design, these features may greatly influence and improve the output of your model's prediction skills. AI techniques can help in automating feature engineering by locating critical factors and creating new data features that are not necessarily obvious.
By doing so, the concentration is on higher-value tasks involving activities such as model selection and tuning, since these can save money and time with the use of automatic function development. Powerful insights to drive AI training engines for actionable insights, and promoting informed corporate decision-making, AI strongly relies on efficient feature engineering.
Model Training
Training the model involves practicing all these techniques of machine learning to train an AI system for identifying patterns in data. This is one necessary stage in predictive modeling for developing a system capable of generating precise views to help an organization predict trends and take remedial measures in time.
The training procedure requires enormous datasets and iteratively modifies the design to increase its power, making sure that the AI learns from experience in data-driven decision-making for new, unforeseen circumstances. Similarly, this iterative modification could be related to the methods like hyperparameter tweaking and cross-validation toward the optimization of model precision.
Businesses can shrink the time to actionable insight AI-from data collection to rapid model training and deployment for real-time analysis. Thus, with data-driven approaches, businesses could adapt to moving market conditions more quickly than the competition.
Prediction and Inference
Once a model is trained, it can be used to reason and predict. AI programs can look at new dates and predict outcomes based on patterns they've uncovered. For example, a trained model might predict sales patterns, client attrition rates, or the most effective marketing initiatives.
These forecasts can also provide informative insights that enable the companies to pre-empt and manage potential issues proactively, such as identifying clients who are likely to leave well before they do. Besides, the ability to predict trends may also enable business owners to reorganize better and change plans to meet altered consumer needs.
In the final analysis, utilization of predictive insight data analytics enables businesses with data-driven AI decision making to strengthen their edge over competitors.
Validation and Evaluation
Both validation and assessment are important to ensure the reliability of AI model behavior. This includes testing these models on fresh data to verify correctness and make the required corrections.
Close monitoring should be done to ensure that the AI models will keep on working as time goes by. Setting appropriate performance evaluation measures also facilitates the early detection of any potential issues for timely remediation.
Models can be improved in efficacy and conditional flexibility by updating and retraining them routinely with new data. Finally, proactive validation and monitoring build confidence in AI systems and inform organizational decision-making about AI.
Deployment and Monitoring
A model goes into production once it is trained, verified, and evaluated. During this phase, its efficacy is tested under dynamic conditions, marking an important turn away from theoretical performance toward practical applicability.
Real-time performance monitoring is necessary to ensure that the model continues to provide accurate AI insights. This will enable companies to realize any degradations in accuracy or relevance over time. This process can be automated using AI technologies, which will alert companies on issues that could compromise the accuracy of the forecasts.
Teams would go on and enhance what was optimized by the model through rapid editing for continuous improvement because the evaluation about shifting trends provides data-driven insights. Businesses applying feedback mechanisms stand a chance to expedite continuous improvements with a general rise in corporate Artificial Intelligence solutions' effectiveness.
Advantages Of Using AI For Generating Data Insights
AI powered insights deliver competitive advantages by identifying emerging opportunities and potential risks before they become apparent. Most firms are incorporating AI-driven technologies into their AI to make better decisions, as AI offers a number of advantages in terms of insights of the data. Following are some of the main benefits of data-driven decision making:

Advanced Data Analysis
It may be understood here that the volumes of data, driven by Artificial intelligence in their decision-making can be done so much quicker than by people and certainly more accurately. This would potentially enable companies to extract deep insights into sophisticated and intricate databases, sometimes exposing hidden trends and patterns invisible to a business. The effectiveness can often improve incrementally through the adaptation of machine learning approaches that cater to new situations and data analysis.
AI can also collate data from numerous sources and draw a comprehensive picture with regards to customer insights into AI behavior and corporate operations. Companies can use AI for the type of insight data analytics in real time that's vital for industries that need to come up with swift decisions that would give them the edge over their competitors and promptly meet customer demands along with the market changes.
Predictive Capabilities
One of the most powerful features of this AI is forecasting the result of future events from past decision-making. A business can forecast sales, shopper patterns, and emerging industry trends by implementing forward-looking analytics.
For example, retailers can maximize their stock room administration by making use of AI to predict which merchandise will be in demand over the coming months. AI can identify and analyze AI preference samples in customer insights for new trends and purchasing habits that give companies a competitive edge over others.
In addition, this AI insight will provide the business with the capability to make informed decisions about promotions and marketing plans by ensuring that the right demographic is reached. Finally, leveraging prediction insight data analytics drives proactive access to resource allocation and business planning with improved operational efficiency.
Automation and Efficiency
AI automates the most time-consuming processes, such as data cleansing, feature engineering, and visualization, within data-driven decision-making processing and analysis. It saves not only time but also reduces human errors; thus, results become more accurate and reliable.
In addition, companies can have more effective resource utilization by automating mundane operations and freeing their resources to work on higher-level strategic projects that will drive growth. This further develops the overall system efficiency, since workers are relieved from repetitive duties and can think in more creative and analytical ways.
In the end, the integration of AI into these processes inspires an innovative culture and allows companies to respond promptly to changes in the market and customer needs.
Improved Decision Making
AI helps organizations make wiser purchasing decisions through the provision of accurate and helpful information. With artificial intelligence insight, businesses can act with confidence, whether optimizing the operation of the offer chain, finding new market possibilities, or determining the best marketing plan.
These insights help businesses stay ahead of the pack through better business performance and an innovative culture. Additionally, businesses can react faster to the request of clients and market changes since they are dependent more on decision-making AI, helping them stay relevant in a business climate.
This relevance is compounded in today's data-driven environment by the fact that increased and better decision-making AI eventually means increased profitability and a stronger market position.
Scalability and Flexibility
Because of great scalability, AI-driven insights are able to handle huge datasets and easily scale with a company. With the growth in business, while data accumulation is growing, AI systems are able to scale and bear the ever-growing load of data-driven decision-making with ease-performance remaining constant while needs rise.
AI models are equally very versatile; thus, easily adjustable to new data sources and changing business environments. That flexibility provides speed to change businesses on emerging client and community trends. Besides that, knowledge earned is augmented through the capability for seamless inclusions of more data-driven decision-making points to yield even better decision-making AIs.
More importantly, this level of scalability will also set companies up for future scaling and efficiently leveraging their data assets as those continue to change.
How AI Insights Help Businesses
Artificial Intelligence insight is potentially expected to bring evolution to various fields of industry. Therefore, some exemplary instances are identified explaining how firms spanning many industries harness this futuristic technology, which would also get an edge for competitive advantage:
AI Insights For Automotive
Application in the auto-industry with analyzing data regarding sensor and GPS, Client satisfaction Survey-based decisions which have been done so for decision-making by taking refinement into manufacturing to raise safety along and pleasantness while driving:.
With the machine learning algorithms, manufacturers can observe trends in the performance of the vehicle and send proactive maintenance alerts showing the drivers possible problems well before these have turned major.
By offering customization in the car's technologies to driver preference, AI-powered insights might enhance consumer happiness and loyalty in a gradually growing competitive market.
AI Insights For Banking
Banks use Artificial Intelligence insight to analyze credit risks, fraud, and personalize client service. Banks can find suspicious behavior through the analysis of transaction data and take necessary steps to prevent it. This will not only protect the institution's assets but also build consumer trust.
Besides, because the personalized recommendations are in line with each client's financial goals and preferences, AI-driven insights can enable banks to provide consumers with personalized investment advice, thus improving client retention and loyalty.
Greater levels of client satisfaction and retention due to this level of personalization can, in turn, positively impact a bank's bottom line and increase its competitive edge in the market.
Data-driven decision-making in banking also allows them to continuously learn from consumer interactions, whereby they streamline their strategies and adapt to the shifting market at an increased pace.
AI Insights For Call Centers
The potential of AI to measure consumer interactions for contact centers and speed up their response time has totally revamped customer insights AI service. AI is capable of monitoring and analyzing consumer queries across various touch points through advanced algorithms and thus providing companies with vital information about customer preference and behavior.
With AI-powered sentiment analysis, businesses can proactively solve issues regarding common pain points in consumers. The organizations will understand the emotional tone in client communications and thus be able to personalize their responses and solutions better.
With greater satisfaction around the performance improvement in customer insights from AI, better-performing call centers also create avenues to long-term business success and loyalty. Again, this in itself would not stress human agents since it actually will help release a number of other tasks a person is burdened with, concentrate on complex calls needing individual attention, and make service quality richer as well.
AI Insights For Finance
AI is applied in finance to predict economic trends, assess stock market data, and optimize investment portfolios. Real-time analysis of big datasets by AI-driven insights can show very profitable trading opportunities.
This improves investment returns and helps traders and financial analysts make better decisions. Additionally, machine learning algorithms can adapt to changing market conditions, so over time, predictions are more accurate.
The AI can find patterns and connections, especially in conventionally data-driven decisions, that human analytical techniques might never notice. Consequentially, financial institutions have moved to increasingly welcome the technology of AI as one way to enhance AI decision-making processes and stay competitive within a fast-changing market.
AI Insights For Government
With governments using AI, governments can further develop and enhance government services. Analyzed data may provide indications on the decisions for security, public health, and traffic management in light of the best resource allocations and policy decisions.
For instance, governments can be proactive by using AI-driven insights to predict the spread of diseases. In addition, AI technologies can enhance urban planning by analyzing population density and movement trends, ensuring infrastructure building meets the needs of an ever-growing population.
Moreover, in law enforcement applications, AI can even provide better utilization of staff, identification of hotspots of crime for a wider protection of public space. Data-driven decision-making at all levels in the government would likely spur a lot more citizen engagement and trust in public institutions, given that accountability and transparency are advanced where the government utilizes AI.
AI Insights For Health And Pharma
AI insight is used in pharmaceutical and healthcare industries to optimize clinical trials, improve patient care, and speed up drug development. AI will be able to predict disease outbreaks, health concerns, and treatment options through the analysis of large volumes of data on patients. This enables early and focused treatments.
AI is accelerating the identification of potential drug candidates in the pharmaceutical industry, considerably shortening the time it takes to market with greater efficiency in R&D. The use of AI could hasten clinical trial processes through qualified person identification, which will be matched according to pre-set criteria; it can further improve the precision of results while cutting down testing costs.
Therefore, such development in two ways brings benefits: better outcomes for patients and a decrease in treatment costs for healthcare systems, thus making more people afford access to certain medical procedures. In any case, AI application to subject areas means a breakthrough toward patient-oriented, data-driven solutions in healthcare.
AI Insights For Hospitality
AI has been inducted into the hotel industry to manage their operations more efficiently and to further develop visitor experiences. This, through machine learning algorithms, lets AI analyze booking information, remarks on social media, and consumer reviews for patterns and preferences.
In return, this enables hotels to serve their customers' needs in the best way and thus ensure that the customers enjoy their stay. This will also facilitate greater efficiency and lower operating expenses in the hotels by using AI-driven insights to estimate demand for personnel.
Equipped with AI in their marketing strategy, hotels can create targeted campaigns to appeal to very specific client demographics, which will raise the level of engagement and reservations over time.
AI Insights For Quantitative Trading
In quantitative trading, the AI systems go through market data to identify trends and execute transactions at the most opportune moments. This approach utilizes machine learning models capable of handling large volumes of data-driven decision-making and responding immediately to changes in the market.
These models can adapt their strategy to ever-changing market conditions by continuously learning from past data-driven decision-making and market swings. Additionally, traders can build complex trading strategies that best fit specific market conditions, thanks to AI-driven insights contributing to the enhancement of decision-making AI.
In the end, this advanced technology helps traders cut down on risks and maximize returns, hence more reflective and calculated investing AI decision.
AI Insights For Market Research
Artificial Intelligence enhances the process of data-driven decision-making in business, processing, and reporting. Artificial Intelligence would enable companies to track competition, watch brand reputation, or even gauge consumer insight analysis results. AI:.
This will subsequently enable the companies to make informed AI decisions on marketing, strategic planning, and product development. Besides, real-time processing of massive amounts of data by AI provides businesses with fast insights that may strongly influence how responsive they are towards market trends.
Businesses can find hidden patterns and connections that may not be so obvious by conventional research approaches using sophisticated algorithms. In the end, AI use in market research increases productivity and provides an opportunity for companies to be one step ahead of the competition with timely adjustments in accordance with changing customer preferences and market conditions.
20 Tools For AI Insights
Artificial Intelligence insight has been developed using various kinds of special technologies that automate data-driven decision making, processing, analysis, and visualization. A comparison of 20 of the most well-known AI technologies for creating insights in industries can be found below:
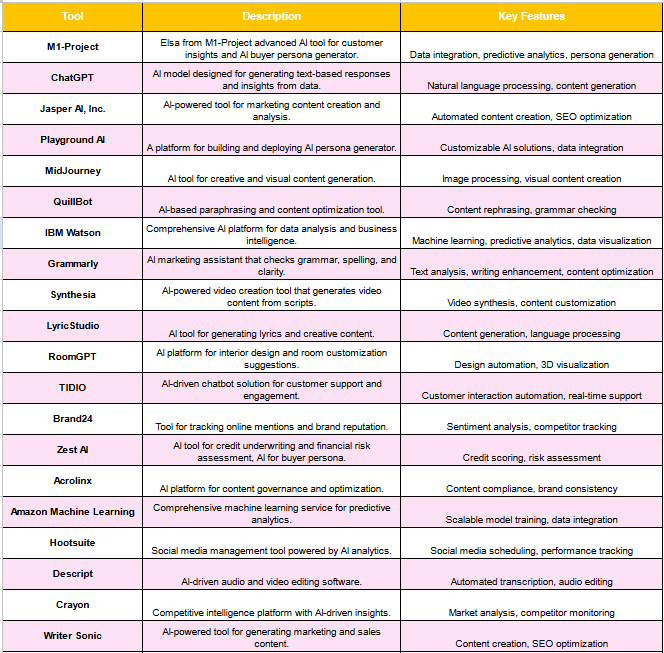
Key Takeaways
Artificial Intelligence insight is going to be one of the essential elements of the modern company plans. They would help businesses to make better judgments and forecast future trends and analyze the data in an effective manner.
AI-driven insights technologies have a lot of advantages: speed, accuracy, and scalability are a few that automatically come with it for complicated data-driven decision-making activities. The following would be some of the important lessons on ways AI might enable data-driven decision making-AI:
- Artificial Intelligence insights speed up data analysis: AI technologies can process massive volumes of data within very short periods and uncover patterns and trends that could not be recognized by traditional approaches. This makes it possible for companies to respond much faster to the needs of consumers and to market fluctuations.
- Improved Decision-making due to Predictive Capabilities: Artificial Intelligence's prediction capability allows companies to strategize and make decisions with a more logical approach. Predictive analytics, especially in retail, healthcare, and financial domains, helps correctly anticipate consumer behavior and market trends for advantage;
- Efficiency is improved by automation: AI permits companies to be in a better management position of resources and focus on important objectives by automating repetitive data-driven decision-making processes in business, reducing the chances of human error plus operating costs.
- Flexibility and scalability: AI systems can grow with the company, handling growing data volumes and adapting to include new data sources. Due to this, they are flexible and future-proof, which is very important for companies hoping to succeed over the long haul;
- Industry-agnostic AI insight: AI insights are changing the way companies do business across industries such as healthcare, banking, retail, and government. Driven by insight technologies, businesses can drive revenue, improve client experiences, and enhance operations using AI-driven insight technologies.
The Future Of AI Adoption
With increasing development of the technology, AI will continue to become more important in data-driven decision making. Following are some of the patterns that may shape the future of AI adoption by organizations :
Increased Integration of AI in Core Business Functions
On the business side, AI has increasingly been used in operations related to marketing, sales, customer service, supply chain management, and human resources. As more organizations embrace AI, the isolated AI solutions will give way for fully integrated systems using data-driven decision-making in business from several business divisions.
It will enable businesses to think at a deeper level about their operations and to make more valid strategic decisions.
Greater Emphasis on Ethical AI and Data Privacy
As AI becomes mainstream, so does the concern for data privacy and ethics. Businesses will need to implement mechanisms that ensure AI systems are fair, transparent, and accountable.
The data privacy acts such as CCPA and GDPR will also have to be followed by businesses to maintain the same level of customers' trust. Ethical AI will be one of the key differentiators while building long-term client relationships for companies trying to do so.
Advances in Natural Language Processing (NLP) and Machine Learning
Capabilities around AI-driven insight solutions will be further developed through natural language processing and machine learning, making it easier for firms to draw valuable insights from unstructured data in the form of text, photos, and videos.
This will open new avenues for sectors reliant on the analysis of social media conversations, consumer reviews, and other forms of qualitative information. AI technologies will make a deeper understanding of sentiment, context, and subtleties possible.
AI-Powered Personalization
One of the most exciting forms of application for AI insights is personalization. As companies learn data-driven decision-making in business about customers, AI systems can give more hyper-personalized experiences to satisfy the unique tastes and desires of every customer.
AI-driven insights customization supports a company in increasing consumer delight and loyalty via everything-from-product recommendations to targeted marketing campaigns.
Expansion of AI in Emerging Markets
Artificial Intelligence insight is not only being adopted by developed nations. Emerging nations are also understanding how AI can help boost economic growth and enhance corporate productivity.
SMEs in emerging economies can compete on a global level with more affordable AI solutions and cloud-based platforms. Due to the democratization of AI, many industries will be widely adopting AI technology.
Collaboration Between Humans and AI
AI will enhance human capabilities, not replace them. In the future, AI adoption will be about tighter collaboration between humans and AI, where humans will focus on strategic, creative, and complex problem-solving tasks, while computers will perform mundane and data-intensive tasks.
Because of this synergy, businesses will have improved outcomes and innovation.
Conclusion
Artificial intelligence findings are changing businesses' approach to data-driven decision-making for reasons such as saving time and effort in business, creating customer experiences that are next to none, and increasing revenue due to automation in data-driven decision-making analysis. So long as technology within the area of AI keeps advancing, businesses shall have the might of seizing newer opportunities and adeptly solving emerging challenges.
However, business-ethics must be rendered an intrinsic part of AI itself, and security and privacy concerns should be attended to in data-driven decisions if businesses are to gain full benefits from AI. On various aspects, the seamless integration of AI into the integral components of the company is going to be the future for AI-driven insights, thus allowing faster, smarter, and better decision-making AI.